FAQs
About AI
How do AI, ML, DL and Computer Vision relate?
Artificial Intelligence (AI) is the term used to define the general capability of a “machine” to do a task considered “smart” or “intelligent” – the “machine” can be hardware or software. While intelligent machines have been around for hundreds of years, more recent and realistic definitions of AI refer to systems that mimic human decision-making, which incorporates consideration of multiple, parallel data streams simultaneously.
Machine Learning (ML) is a technology by which machines use historical data to train themselves to behave in a certain way; thereby, Machine Learning enables more rapid growth of AI.
More recently, a more evolved method of ML has emerged, known as Deep Learning (DL). DL incorporates artificial neural networks to mimic the functioning of the human brain, thereby enabling more efficient learning.
Computer Vision (CV) bridges ML and DL to help machines use historical data to perform human-like identification tasks using vision.
What specific business issues can AI/ML/DL/CV solve? How can these technologies be successfully implemented?
A 2020 MIT survey revealed that over 60% of the manufacturing industry uses AI to improve processes and product quality, build efficiency into their supply chain, and enable areas like asset management and predictive maintenance.
Drilling down into these applications, some of the use cases are:
- Preventative maintenance: Using data from various sources like IoT systems, production control systems and gauges, inspection data, and human input, along with data collected over time to learn about historical asset changes and predict the future state of assets to perform preventive maintenance. AI data from these sources can identify corroborative patterns and build highly accurate models of future states and risks. This helps in planning for early repairs before accidents happen and enables planning for spares and repairs to build efficiency in the entire procurement process.
- Situation-aware robotics: Traditionally, robotics was, and still is, used to automate assembly line production and accurately implement designs into precision manufacturing tasks. Today, the industry is exploring robotics for highly repetitive and high-risk tasks. More current AI capabilities allow robots to adapt to changing situations and environments and continue to perform tasks, be it manufacturing or process inspection and monitoring. With AI, industrial robots can monitor their accuracy and performance and train themselves to improve. Additionally, robots can be equipped with machine vision to enable more precise mobility in complex or random environments. More recently, robots are being programmed to work alongside humans in tasks that cannot be fully automated.
- EDGE analytics: Grouping the capture, analysis and tracking of asset and component degradation to speed up the identification of failure risk and take early action. EDGE also helps improve the efficiency of data flow across different layers of an enterprise system while speeding up response. EDGE is typically used in product quality analytics and component level sensors to detect potential failures; it can also be used in computer vision-related applications to detect risks and, in the workforce, wearables to monitor health and safety.
- Inventory and Supply Chain Management: AI can help plan inventory, spares and supply.
- AI-powered Digital Twin: Create and maintain real-time digital replicas of physical assets and keep track of all live data. Digital Twin use additionally enables deciphering of cross-system data, variables, variations, risks, bottlenecks and, simultaneously, progressive asset changes and performance, as well as workforce safety.
When it comes to successful implementation, industries require the obvious technology, the digital discipline, and the digital framework information to achieve successful outcomes. Most industries treat AI as a technology tool they have to implement.
How will the live digital twin/metaverse/digital avatar transform the maintenance and inspection industry?
The metaverse is an immersive, interactive, virtual medium where real-time digital representations are made of the physical world, and digital/ computerised actors can interact with them.
In the traditional industrial world, the dynamics of these interactions become more complex in terms of quality, safety, environment, security, efficiency, cost, accuracy and other operational decisions taken by the asset manager. These decisions are traditionally made based on complex data inputs from different and disconnected data sets, many of which are static, manually captured, are not necessarily current in time and often reactively captured after an event.
The maintenance and inspection industry has traditionally incorporated manually driven processes, with some technological adoptions toward automation. The metaverse enables full digitisation of processes, including the maintenance and inspection process, by merging disparate physical systems and data they generate and creating a near-real-time virtual representation of the process.
By using the metaverse or the digital avatar of the industrial operation, the asset manager can have a current live view of the asset as it is right now, with data that is consolidated and corroborated across different data sets enabling the asset manager to make predictive and preventive decisions on the asset maintenance function.
Keys Benefits for the Maintenance Industry:
- Safer training environments for staff where they can experience the physical asset in the virtual environment before interacting with it
- Safer working environments by having an integrated real-time, virtual view of the asset to assess safety, and take necessary action, whether it be the deployment of remote actions using drones, robots or emergency triggers.
- Improved predictive maintenance by simulating and extrapolating real-world data in a virtual environment, knowing in advance if any action is required to prevent critical events.
- Improved efficiency by reducing time, costs and accuracy of findings, along with better informed corrective actions resulting from a more universal and unified decision-making system.
- The ability to fine-tune production processes and systems and align them with the demand means that the supply chain can be efficiently operated upon in real-time or as close as possible to real-time.
- The ability to monitor assets and their impact on the environment (which will necessitate the interaction of the digital version of the industrial asset with the digital version of its environment) and keep track of mandates and environmental goals to achieve sustainability goals.
The Company
How does oceans.ai contribute to the ‘net carbon zero’ objective? How can AI create a greener (more sustainable) industry?
Every industrial asset, be it existing or still to be manufactured, has an environmental impact. A critical challenge facing industries is maintaining, repairing and replacing assets at the optimum time to minimise environmental impact.
Industries need to find optimum ways to take preventive action on an asset before it fails or before it needs to be replaced. Three factors drive this:
- The ability to identify the assets’ current status across its different parameters of operation, condition and environment in real-time.
- The ability to use historical data to identify progressive changes to the asset, its components, and when repairs/replacement are required, along with insights into optimum timing for repairs/replacement.
- The skill of knowing when the right time to replace an asset is person-dependent, and it is accrued over time. If the person leaves, the skills and human insights are also lost.
With aggressive carbon-neutral compliance now at the forefront of many businesses, the need for intelligent data systems that provide insight into the environmental impact of industrial assets is growing. Companies need technology solutions to:
- Measure asset environmental impact.
- Take corrective action on assets that are negatively impacting the environment.
- Be able to predict assets’ impact on the environment well before any adverse or permanent effects.
AI is the cornerstone of these technologies, but without the workforce, AI is nothing but a dead tool. The workforce knowledge, combined with AI, will enable industries to achieve environmental goals. Oceans.ai solutions are designed to help industries achieve these goals through Industrial Intelligence in a systematic manner while building inclusivity and sustainability of the workforce and their knowledge of the transformations these industries require.
How sustainable is Oceans.ai’s technology?
Oceans.ai is committed to the UN’s sustainable development goals. With this in mind, our impact on the industry and these goals are twofold:
- We aim to deliver solutions to sectors like energy to help them achieve sustainability goals through predictive and preventive maintenance. For example, we are currently working to provide a comprehensive AI-powered, end-to-end wind turbine inspection solution that will help improve the running time of turbines while reducing the risk of failures and breakdowns. We are also working with the traditional energy industries to help them reduce their environmental impact and carbon footprints by creating Digital Twin solutions that simultaneously monitor assets and the environment to provide valuable insights.
- As a company, we know that AI also creates a carbon footprint. Oceans.ai is committed to using efficient architecture, green infrastructure and technology to deliver our solutions. Oceans.ai focus on working with like-minded partners and suppliers to ensure we meet and exceed sustainability goals as we grow.
How would you assess the intelligence of AI?
Just as Intelligence is not absolute, AI, too, is not absolute. Therefore, no single benchmark can help us measure the intelligence of AI (until humans find a mechanism and means to define “AIQ”). Until then, the measurement and success of AI will be bound by the outcomes we manage to achieve in AI applications. While there are some basic methods and mechanisms to measure AI accuracy and confidence, the levels of measurement we will need to apply are nowhere close to assessing the outcomes we seek to achieve.
AI is intelligent to the extent that it can process large volumes of data in a tiny measure of time, identify patterns and contexts across different and disconnected data sets, can consistently repeat tasks without fatigue, can corroborate data sets that seeming appears unrelated, and can predict outcomes based on historical information and trends. But to be able to make the balanced decision-making that humans do, sticking to the right approach of assessing the risks, measuring the pros and cons and evaluating emotional and empathy-related decision-making, balancing IQ with EQ is something that AI will still need humans to be involved in.
This is precisely why at oceans.ai, our system processes and workflows are designed keeping the concept of human-in-control, where the final assessment, decision and intervention will always be human.
Our Solutions
How do I train employees to co-exist with mobile robotics and AI?
Oceans.ai believe that AI is just that: Artificial. It becomes real only when harnessed and applied by humans. Furthermore, as we know, in the real world, there are very few absolute right and absolute wrong choices. Every choice is a shade of grey and based on what is more right by balancing the benefits versus the risks.
With this principle in mind, every solution we choose to build at oceans.ai incorporates the human intelligence envelope over the application of AI.
AI is to be used not as a tool to replace the workforce but to increase efficiency and improve workforce functioning so that the overall outcome benefits all stakeholders. The emergence of ‘Cobotics’ (robots that co-exist with the human workforce) bears testament to the AI industry’s awareness of the importance of programming robots and AI to co-exist with the workforce. It is the workforce that is key to the success of AI.
What exactly is an autonomous mobile robot/drone with oceans.ai solutions? How is AI used in robots?
Autonomy refers to the self-governing ability of a person/ group to choose a course of action. In robots, it essentially means the ability of the robot to deal with changes in its operational environment, over extended periods, without human intervention. When it comes to oceans.ai’s solutions, it essentially means:
- the ability of the robot or the drone to perform the act of data gathering autonomously, without human intervention
- the ability to transmit data to the cloud-based platform for processing autonomously
Once the cloud-based platform receives data, the rest of the AI-based processing takes place without the robot. AI enables the robot to identify its environment and changes therein and interpret the differences, identify corrective/responsive action and, if required, immediately trigger an emergency response.
What type of AI is oceans.ai currently using? In the future?
At oceans.ai, our use of AI focuses on helping industries achieve the highest possible safety, sustainability and efficiency levels. Towards this, we leverage all forms of AI required to address industrial challenges. While a significant portion of our effort is focused on Computer Vision based AI, we intend to integrate data-driven AI for IoT, robotics, Edge and other industry 4.0 sources of information.
How do you feed machine learning? What data do you use?
At oceans.ai, we believe humans must be a part of the machine’s learning process, including how AI learns and improves itself over time. The human is regarded as an AI mentor of sorts. And because of this, all our AI systems are designed with the “on-the-job-machine-learning ” process, where AI learning sits in the middle of the live industrial process and is mentored by experts in the field in real time. The live data generated through the industrial process is fed into learning, and, in due course, the learning is applied and then verified by the workforce, thereby perpetuating the loop. At all times, the workforce controls the AI learning process.
In both DeepDIVE and INSYD AI technologies, AI learning is part of the platform and continuously learns from the digital process and the workforce’s feedback loop.
Our Policies
How is my data safe on oceans.ai platforms? How does oceans.ai protect AI from hackers and security breaches?
Data security and privacy are at the core of the oceans.ai platform and data framework. Oceans.ai respect the proprietary nature of client data and, thereby, the importance of keeping data control in the hands of the company to which it belongs.
Our platform is deployed on Google Cloud infrastructure, and we leverage the safety and security provided by this platform to address the safe access mechanisms of our solution. We also extensively use secure transactions and proactive data access to transmit information across the internet.
Prevention of our systems and platform from security breaches is a continuous set of mitigating measures thatoceans.ai is involved in to ensure the data processed on our platforms are secure. We use the best-of-breed technologies and processes to ensure these are constantly updated and mitigated. We work closely with our platform providers, putting in place our strict controls on user access, admin access, data access and model training methods to ensure that only those authorised to access them can do so. We are constantly making our teams aware of security measures employees must take to keep themselves aware and prevent phishing or malware attacks that may potentially breach their desktop security, putting the organisation’s and our customers’ data and information at risk.
We will continue to improve these efforts as data security is one of the critical pillars of our platform’s philosophy. We will do the necessary to keep that pillar intact.
Please get in touch with us if you have specific queries or concerns about security.
How can I retrieve my data (and what won’t I be able to take away) if I decide to use another platform?
At oceans.ai, we believe your data belongs to you. So, whenever you choose to leave the oceans.ai platform, we provide all raw and processed data in a structured format to enable clients to transfer and utilise information in any other system or platform.
Contact us today for a Demo
We can help you assess different strategies to reduce costs, improve productivity and become more impactful on the environment.
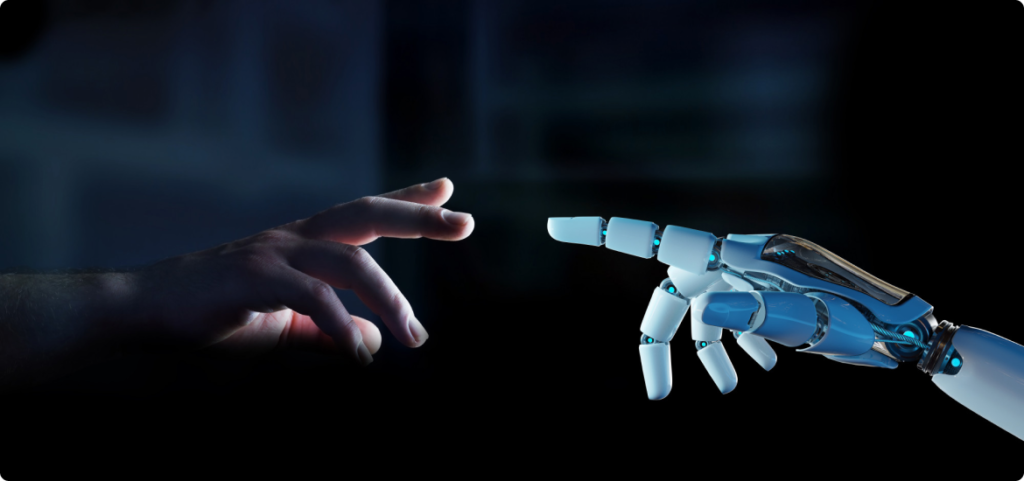
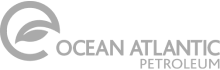

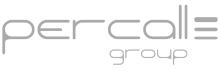
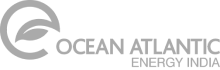
