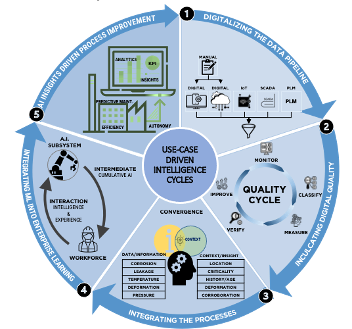
Continuous on-the-job learning at the core of industrial AI/ML
In 2019, IDC predicted that by 2021, 75% of all digital transformation would incorporate AI initiatives. While this has held chiefly true, industries see that adopting AI is more than just a factor in acquiring the technology as per their digital efforts. AI is turning out to be a transformative revolution akin to a cultural revolution in industrial operations.
One of the key factors to remember in this transformation is that just as the operating environment is continuously changing, workforce intelligence (and thus the supporting artificial intelligence) needs to evolve constantly. And this requires a transformative approach towards the implementation of AI in industries.
As with all transformative revolutions, grass-roots-based initiatives need to be factored into the fabric of operations to make AI and ML successful initiatives. More importantly, it is also to be noted that this kind of transformation takes time to happen, where AI’s magic bullet will solve all industry’s challenges. AI and ML will be treated as toolkits that will help the operational team and the workforce enhance the speed and accuracy of industrial decision-making.
But we jump the gun. Before even plunging down the AI line, organisations must address some key and critical factors associated with their digital environment.
As stated in an article in www.themanufacturer.com [1]: “According to digital workflow leader Infoware’s independent survey of 1,030 UK-based industrial firms, the vast majority of manufacturers (86%) claimed to be data-informed, and 76% said that they trust data enough to complete tasks, this is despite most of them relying still on disparate legacy systems.”
Industrial asset data now, and how should it be?
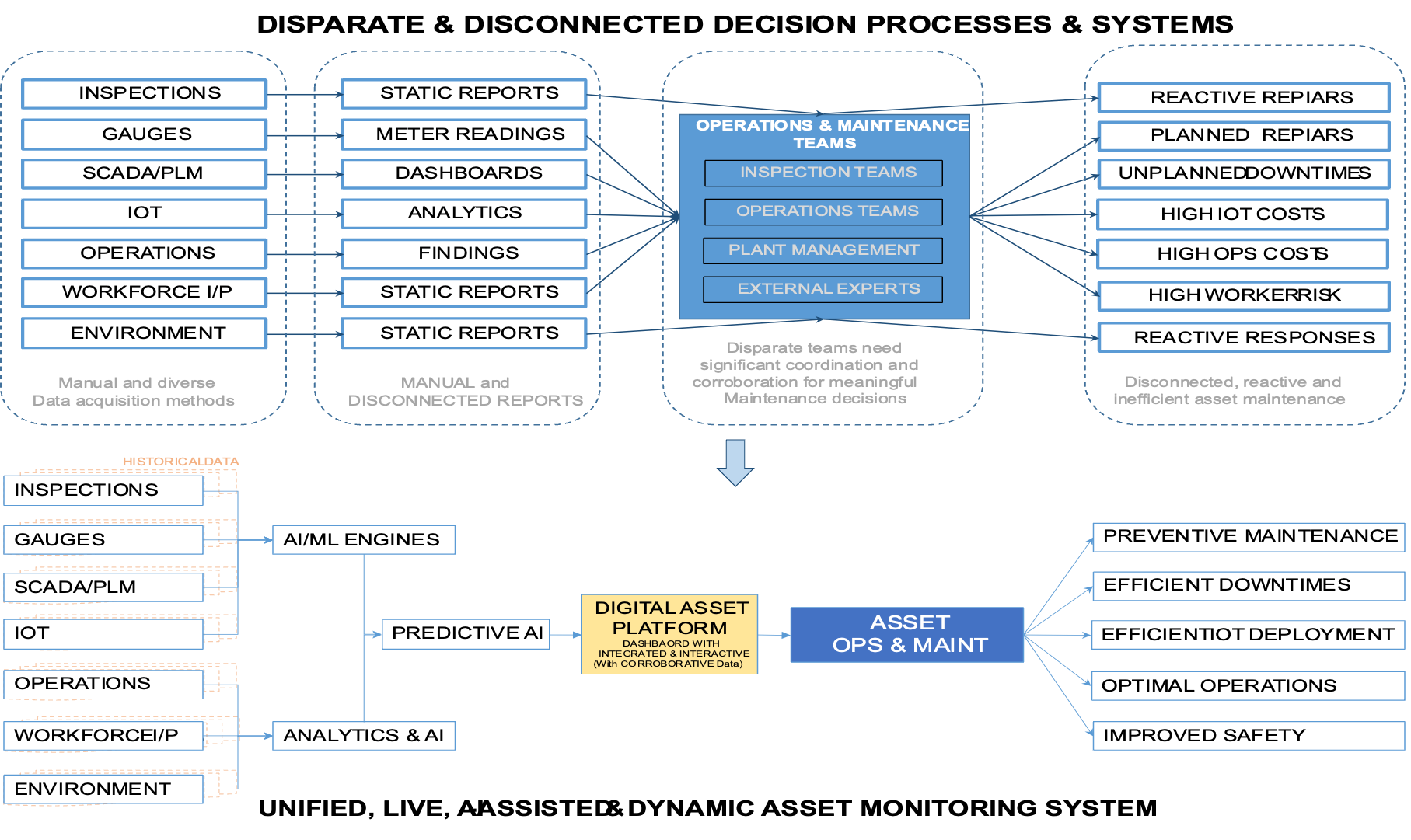
Most industries have legacy systems, siloed and disconnected and, more often than not, in spreadsheets. These systems are then used to generate static reports from different operators and providers in different formats.
Using these reports, human experts find connections and patterns between these disconnected systems to draw intelligent decisions from them. These decisions are usually retrospective, thus taken post an event.
Even here, multiple layers of human experts analyse each area of specialisation and give their opinions based on limited data visibility.
Furthermore, by the sheer volume of data that has to be tracked, assessed and analysed, the human mind can’t bring them all together and provide intelligent insights into the assets/operations status and condition. More importantly almost impossible to make an accurate predictive judgement without a deeper understanding of corroborating data sets.
A key example of an industrial operational process is asset inspection and monitoring. This keeps the plant and the asset running consistently and optimally.
The journey from “Disparate & disconnected decision processes and systems” (above) to a “Unified, live, AI-assisted & dynamic asset monitoring system” (below) is a series of incremental steps that need to be systematically implemented to achieve its intended outcomes.
The recommended approach to Industrial AI.
Industrial processes are complex and built over many years. They cater to various fundamental paradigms associated with the business process, the outcomes, the quality, the costs’n’profitability and regulatory expectations of the industry. These are very intricately woven to build successful outcomes for the business.
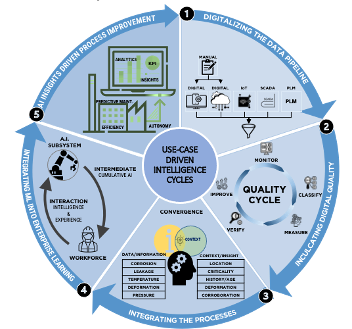
Implementing artificial intelligence into such an intricate system can influence all these fundamental paradigms significantly, and the successful or negative impact of its influence depends on how this technology is adopted in the industry. A systematic and cyclical method of incrementally implementing AI would deliver the best results for the industrial adoption of AI.
Each stage and step is designed to improve a key brick within each operational process. At an execution level, these vital steps must be put into the repetitive implementation cycle.
- Digitalizing the data pipeline
- Standardise and automate data acquisition for each use case. This is unique and specialised for each use case; thus, data acquisition needs to be fine-tuned to streamline the entire data pipeline for AI learning, and since context is essential to AI learning, capturing the context in the data acquisition is important. Standardising the digitalisation of complex data-gathering processes can be quite complex in an industrial environment, especially without losing context.
- Integrate the EXPERTS (workforce) into the transformation exercise from data acquisition to data certification to ensure that Ai learning happens through expert data science and that there is a continuous maker-checker model for AI.
- Inculcating Digital Quality practices
- Make Data quality integral to the business process and drive quality by capturing real-world business data, context and insights: Once the experts are in place, the data quality process must be continuously built into the digital system. Data quality in AI projects is typically seen as a one-time activity in the initial learning process. Context also impacts data quality. This is because while findings can be the same across different contexts, the business operative context and expert’s insights will determine the action that needs to be taken. Take, for example, the ability of a computer vision system to identify a crack or say what appears to be like a crack. While cracks are critical, the level of criticality is based on where the crack is, in which operational component it is, in which stage of the operating cycle it is and so on. Also, not all findings have the same action outcome, and if AI systems have to help improve decision-making, this real-world changing business data, context and quality must be integrated into the process. Without it, we may end up with an incomplete and possibly wrong context AI identification triggering costly operational or maintenance team intervention.
- Integrating the processes
- Through data integration: Now that we have data and all the key stakeholders built into the cycle of continuous gathering, learning and improvement, we need to integrate the data into a meaningful integration across the various sources. Unifying and optimizing the entire data to bring in an efficient and accurate digital reflection of the process is imperative to successful integration. This will involve Identifying the essential learning and supportive data bricks and identifying the data patterns, contexts and classifications that will provide the right digital view of the industrial process and its historical performance. This will be an iterative process that should be evaluated to see which integration and slice of data will effectively digitally represent and track the process.
- Unification of information across all stakeholders (everyone sees the same insights): Now that the process is streamlined, the key stakeholders of the process must be all in the same spectrum of all the connected data sets, insights and information. This is critical, so there aren’t any conflicting isolated decisions that could potentially lead to cross-purpose decisions negatively impacting the industrial process. Using the right tools and visualizations, all the stakeholders see the same insights and corroborating data as the rationale behind the AI logical recommendation. This approach can also drive effective enablement of the maker checker model, so there is no single point of failure.
- Interactive ML in Enterprise Learning
“Most activities at the human-machine interface require people to do new and different things (such as train a chatbot) and to do things differently (use that chatbot to provide better customer service). Organizations that use machines merely to displace workers through automation will miss the full potential of AI. Tomorrow’s leaders will instead be those that embrace collaborative intelligence, transforming their operations, their markets, their industries, and — no less important — their workforces”. – James Wilson and Paul Daugherty “Collaborative Intelligence: Humans and AI Are Joining Forces”[2]
- Process streamlining (with Human-in-control): Accountability of the decisions will still need to be human, even with AI-powered systems. Once the data is organized, it must flow through a process and workflow to work effectively with the correct checks and balances. There is a need for human-based control of the AI’s decision-making process. This is imperative to ensure that the AI does not make decisions that incomprehensible to humans. Human decisions are a balanced choice between various data-driven points and soft factors associated with the various stakeholders. We must keep that power and choice with humans so that we are fully aware of the options and decisions that are taken, especially those liable to bring errors and redundancies into the process. While doing so ML also needs to be a cyclical incremental process: The human learning process too continuously evolves. “Anyone who stops learning is old, whether at twenty or eighty. Anyone who keeps learning stays young. The greatest thing in life is to keep your mind young.” — Henry Ford. Without continuous learning and improvements in human interactions, AI models will soon become outdated. The essential advantage of AI/ML is that continuous learning can be cumulative across the fragmented intelligence in the enterprise workforce.
- Building workflows based on insights
- Build and calibrate the right decisions and actions on insights: Now that we have all the key building blocks in place, we will implement the proper workflows and the right action decisions to be taken on the outcomes provided by the integrated AI. This is a crucial factor. Especially in integrated AI systems where one finding may either lead to an autonomously triggered further investigation or may lead to an action that may change the status quo of the process/operation. These will need to be carefully calibrated and measured with appropriate checks and balances to achieve the optimum outcomes.
.
The above approach to implementing industrial AI is to help support, speed up, make efficient, make safe and improve the accuracy of the human decisions making process. It is not to be and is not practical to design AI implementation to replace human skill sets. Instead, it should complement and make efficient and improve the outcomes of human decision-making. This is the only correct way to implement AI
[1] https://www.themanufacturer.com/articles/74-of-manufacturers-held-back-by-disconnected-data/
[2] https://hbr.org/2018/07/collaborative-intelligence-humans-and-ai-are-joining-forces
Leave a reply