
Business, technology and financial benefit of AI-driven sustainability in industrial Asset Management
Businesses face a tremendous amount of challenges in the current operating environment. With the dynamics of the world rapidly changing and evolving, it is imperative that businesses find the right tools and solutions to address these challenges, and address them well before they manifest into a symptom. The reality of the environment demands that businesses understand the reality, the challenges and prepare an approach to tackling them. Artificial Intelligence (AI) has shown tremendous potential to be a key weapon in the arsenal for businesses to tackle these challenges. The question is how can it be utilised. Here we discuss one such possible application in Asset Management.
Below are the two main factors to take into consideration: the business and economic reality (1) and the climate reality (2).
(1) Maximizing the productive life (and minimizing disruption) of an industrial asset is inherently necessary for the business to improve its productivity and profitability. It is also key for economies to continue to grow and support the global population and the world’s challenges.
(2) Year 2022 – “Projections show global emissions in 2030 will still be roughly twice as high as needed to limit warming to 1.5C”. IPCC. Climate Change 2014: Synthesis Report. “Based on the year 2020, the industry sector accounts for approximately 24% of the global emissions of greenhouse gases”. [1]
Combined, they form what we call the “converging reality”. Simply stated, it is beneficial to the environment that businesses maximise the asset usage (so as not to consume new ressources for new assets). At the same time, they need to ensure that the asset, over time, is not having a negative impact on the climate, thus outstripping the impact of a new or replaced asset.
The converging reality is summarized in the 17 Sustainable Development Goals (SDG). The private industry sector is impacted by a few of them: SDG 7 Affordable and Clean Energy, SDG 8 Decent Work and Economic Growth, SDG 9 – Industry, Innovation and Infrastructure , SDG 11 – Sustainable Cities and Communities, SDG 12 Responsible Consumption and Production and SDG 13 Climate Action. These are directly in the line of sight of industrial economic activities. They need to be addressed effectively and quickly. [2]
The key to unlocking this complex reality puzzle:
Here are the tasks of immediate concern for management in industries : identify their operational areas that affect these SDG, gather the information systematically, be able to measure the impact of the data on the SDG goals and identify the factors that are impacting the slippages and to take intelligent and informed corrective action. Another major task is to know how will the industry strike the right balance and achieve the converging paradigms set in the SDG’s and the business goals.
Many of the SDG’s are very likely at cross efforts with each other requiring industries to maintain the right balance to achieve them. Additionally for industries, amongst all their operational goals and responsibilities, maintaining their industrial asset operations at the optimum balance between sustainable productivity, profitability, efficiency, responsible consumption, safety and achieving climate goals is right up there amongst the top focus areas over the next 20-30 years.
As per UNIDO industrial report 2022[1] ‘Industrial policies should exploit technology “pull” and “push” pressures strategically’ and “Industrial policies should also support the digitalization of manufacturing.” including, “digitalization of monitoring and tracing processes, predictive maintenance and production optimization” as amongst many other factors industries should put in place to be able to achieve these goals.
Today’s industries, while having adopted technology for industrial transformation and automation, including adoption of Automated control systems SCADA & PLM, are still some ways away in bringing all the various complex, disparate and disconnected systems and voluminous information sets from them and make integrated informed decisions on their operations. Furthermore, many industries are putting in place IOT, Sensor and robotics to help the production process.
All these systems and technologies gather large volumes of data. But the process of gathering information and gaining intelligent insights is a combination of manual, tedious activity (Inspections and Asset Monitoring) combined with information technology powered Analytics and Insights. Data from these complex disparate sources, Analytics from business intelligence systems and Big Data systems and digital simulations data are gathered together to identify what went wrong retrospectively.
On finding the reason, corrective actions are implemented to prevent that event from happening in the future. Till something else goes wrong. Furthermore, the same solution is applied to other seemingly similar contexts, without the realization that the factors influencing that context can vary from the first.
The corrective action is either retrospective in nature or is done way too early. In both cases, the impact to the SDG goals can be counter-productive.
AI to the Rescue:
Industrial systems are highly complex systems. It is not just one event that triggers a crisis but multiple factors that influence catastrophic outcomes and these factors change over time and in context.
To address these challenges, industries need to adopt 3 KEY action blocks:
1) Test: Test learning, provide for feedback and feedback loop, continuously improve, keep monitoring and mentoring.
2 ) Learn: Continously learn and improve, corroborate and cross-learn.
3 ) Assimilate: Continuously organise and classify data into meaningful, context-able data sets, gather information and time data systematically, data to be learning friendly.
You see that there is nothing new about these 3 blocks. These are standard process blocks in any learning process. What is new is the power of AI combined with “AI in the loop” (meaning including AI in the human operational process) when applied to the task of industrial data processing. It helps delivering immensely powerful outcomes for the industry.
AI, AI infrastructure, AI Architecture and its evolving technologies have the focus, efficiency, federalisation, expandability and efficiency to deliver the required outcomes of these SGD’s.
In The role of Artificial Intelligence in achieving the Sustainable Development Goals[1], the authors documented evidence of the potential of AI acting as (a) an enabler or (b) an inhibitor on each of the 17 SDGs.
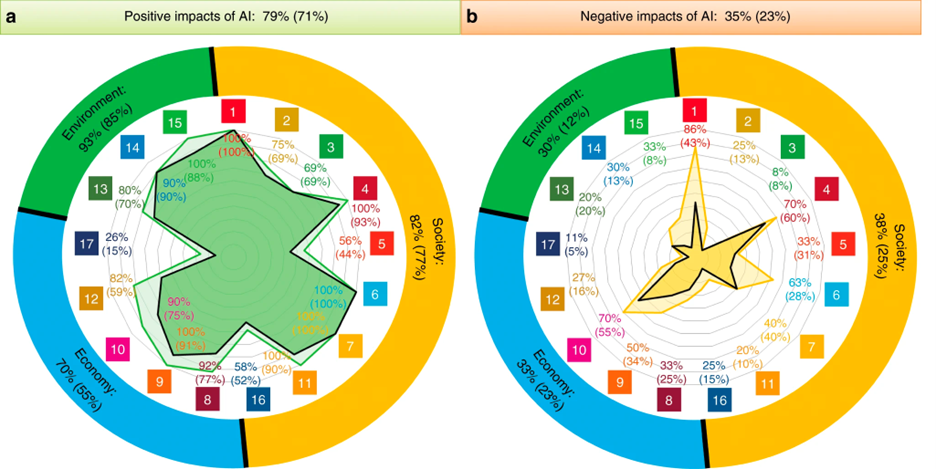
Source: The role of artificial intelligence in achieving the Sustainable Development Goals
Operational & Financial Impact of AI on industry:
AI is also emerging as a new factor of production that can help kick-start profitability and improve cost effectiveness. Across multiple industries, business profitability has steadily declined over the past years, thus eroding future investment and shareholder value. The new period of prolonged inflation we have entered threatens to further reduce profitability. In several industries, the cost of production has jumped by 30 % or 40 % over the past year. This is unbearable.
AI can have multiple application:
– Heavy industries, such as Energy and Manufacturing, usually require large up-front investments. Think the renewable energy sector, for instance. This makes them very vulnerable to the lost revenues associated with asset downtime. Consider wind turbines. Unexpected downtime requires a coordinated effort to source equipment, maintenance staff while cutting into revenue. This can result in one or two weeks of asset downside in case of gearbox failure. Several start-ups are working on an algorithm based on the human immune system aimed at predicting and preventing failures. The algorithm monitors the turbines in real time to detect symptoms and flag any potential problems.
– Canadian Solar, one of the world’s largest solar power and energy storage companies, is working on two projects. The first one is about predictive maintenance – one of the recurring headaches in the energy sector (this is similar to the previous example). In solar energy production, the inverters (which are used to convert the energy produced to the electricity grid) often fail.
Four main factors can cause failure: excessive use, overvoltage and undervoltage, ultrasonic vibrations (which can cause friction and damage inverters) and wear-out failure of capacitors (they have a limited lifetime and age faster than other components, thus causing inverter failure). When this happens, solar energy production can drop by more than 20 % on average.
Using AI, it is now possible to detect anomalies upstream and to predict failures before they occur at the level of the inverters. This leads to higher operational efficiency and significant cost savings. The second one is about production forecasting. Selling power freely and managing it to get a good price can increase the profitability of a solar plant by more than 40 %. Normally, power is sold in bulk to a trader who does this work. Canadian Solar, and other competitors, are looking to get into this business. But they need AI to help them understand how their plans work.
These are only two examples in the renewable energy sector.
AI is seen by the whole industry (including the most polluting sectors) as one of the answers to make profitability sustainable. The year 2020 served as a wake-up call for the industry on the need to move forward into the green transition. This won’t happen overnight. It will be very costly. The firms that will not comply with this new norm (going green, going big) will pay a much higher cost than the ones that will embrace this change. Basically, they will pay higher taxes (carbon tax).
Nowadays, firms can reduce the internal temperatures in buildings and factories, for instance. This is a short-term solution to fight climate change. AI can bring a long-term solution to improve energy efficiency. AI systems are implemented as pilot projects in several countries to manage building energy while maintaining maximum comfort with minimum expenditure. The first results are encouraging. Savings in consumption can exceed 20 %.
At Oceans.ai, we believe the green transition is based on 3D: Digitalization, decarbonisation (electricity efficiency, power-to-heat, power-to-cold) and decentralization (going local). In each of these, AI can play a major role to get the best and faster results. This is what the industry as a whole has perfectly understood over the past two years and is working towards realizing these goals.
Vinod Govindan, Founder and CEO of Oceans.ai, and Christopher Dembik, Economist and Advisor
About Oceans.ai
Oceans.ai is a transformative digital solutions company that is building digital solutions for the industrial world, enabling them to systematically and methodically adopt solutions and technologies to power their AI transformation journey. Visit oceans.ai at www.oceansai.tech or write to us at sales@oceansai.tech to learn more about their solutions and how their offerings can help.
[1] Vinuesa, R., Azizpour, H., Leite, I. et al. The role of artificial intelligence in achieving the Sustainable Development Goals. Nat Commun 11, 233 (2020). https://doi.org/10.1038/s41467-019-14108-y
[2] https://www.unido.org/sites/default/files/files/2021-11/IDR%202022%20OVERVIEW%20-%20EN%20EBOOK.pdf
Leave a reply